Machine learning algorithm could offer urologists a “crystal ball” for predicting VUR
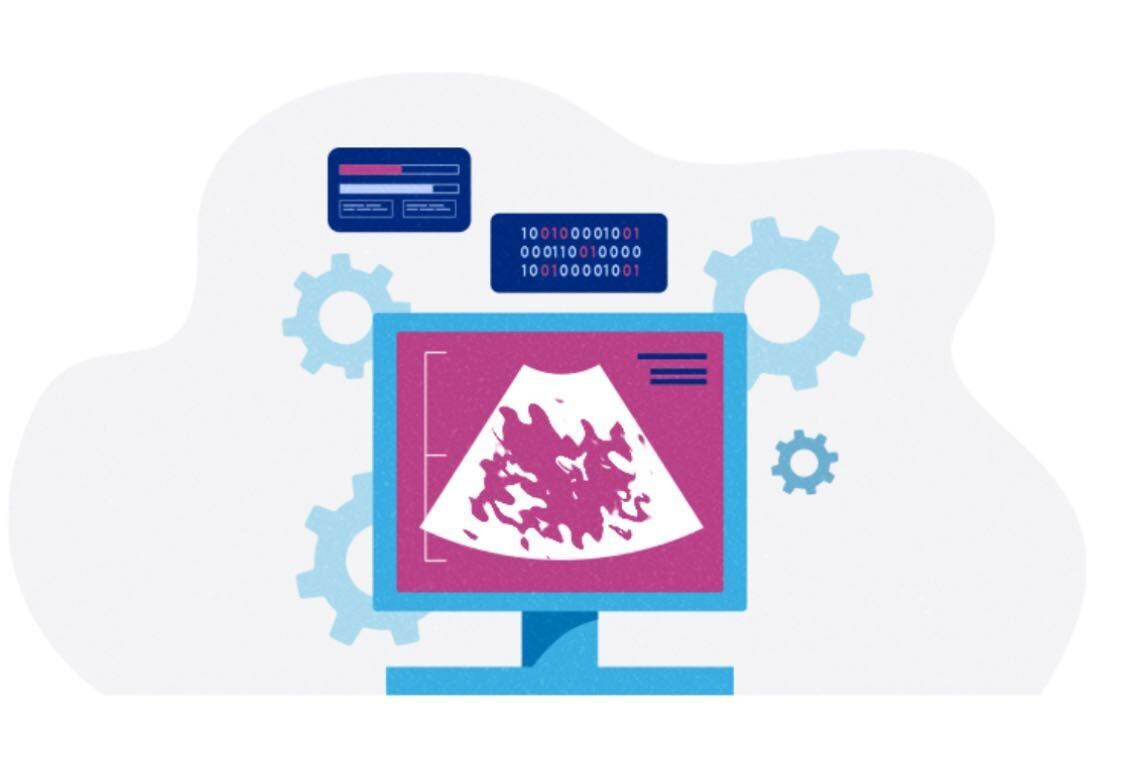
Hydronephrosis is a common congenital anomaly that’s increasingly identified during prenatal ultrasound. Although ultrasound is also the first-line test to screen for hydronephrosis in infants, it is poor at determining dilating vesicoureteral reflux (VUR), one cause of hydronephrosis. A voiding cystourethrogram (VCUG) can better characterize VUR but is more invasive and costlier.
But what if urologists could draw on certain ultrasound findings to predict which patients are more likely to have VUR — and would benefit from further screening with VCUG?
That’s the goal of a new machine learning model developed by urologist Hsin-Hsiao (Scott) Wang, MD, director of Boston Children’s Computational Healthcare Analytics Program, and his colleagues. The model is believed to be the first of its kind for accurately predicting risk of dilating VUR based on features of early postnatal ultrasound.
Robust performance in predicting VUR
Since 2014, urologists have relied on the standardized urinary tract dilation (UTD) classification system to evaluate hydronephrosis in infants. The system uses six pre- and postnatal ultrasound findings to classify the likelihood of UTD severity; in general, the more a kidney appears dilated, the more likely the presence of some underlying anatomical abnormalities. However, this approach can’t provide a specific diagnosis or predict outcomes.
Wang and his colleagues have already developed machine learning models to predict which children with recurrent urinary tract infections — a symptom of VUR — might merit further testing. For a new study, published in the Journal of Pediatric Urology, they sought to develop a model that could reliably predict dilating VUR based on postnatal ultrasound and determine which infants would benefit from VCUG.
To do so, the team reviewed data from 280 infants who had undergone first ultrasound imaging for hydronephrosis and then VCUG to confirm dilating VUR at Boston Children’s. Based on this information, they developed a machine learning model that uses distinct patient and imaging details (such as demographics and UTD classification features) to predict risk of dilating VUR among infants with hydronephrosis. When put to the test, the model’s ability to predict VUR was strong, with an “area under curve” of 0.81 out of 1.0.
More robust model in the works
What’s more, the model is intuitive and interpretable for clinical use, says Wang. “The model can be easily reviewed and utilized in routine clinical settings when counseling patients for the next steps of management for hydronephrosis,” he explains.
The team is currently working on an even more robust model, drawing from some 15,000 patient records to produce an algorithm that hopefully will predict each patient’s future in terms of hydronephrosis: Will it resolve on its own or will the child develop anatomy that needs to be corrected?
“We hope to build a crystal ball for urologists so kids don’t have to undergo unnecessary testing,” says Wang.
Learn more about Boston Children’s Department of Urology and Computational Healthcare Analytics Program.
Related Posts :
-
Drawing on data to revolutionize pediatric urology
At first blush, data analytics — sifting through raw data to discover patterns and draw conclusions from them — seems far afield ...
-
Harnessing the power of machine learning to improve urology care
Urodynamics — a group of tests that evaluate how well the body collects, and then releases, urine — can be crucial for ...
-
Predicting the best treatment for vesicoureteral reflux in kids: The power of machine learning
The result of abnormal formation of the normal valve between the kidney and bladder, vesicoureteral reflux (VUR) can lead to ...
-
Building a bladder-specific Botox
Botulinum toxins are bacterial neurotoxins that also have a range of therapeutic uses. In urology, injections of these potent substances ...